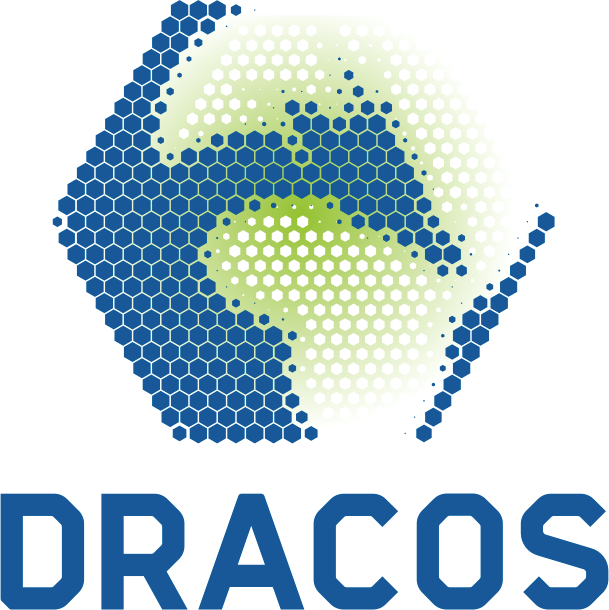
Data-driven analysis of complex systems (DRACOS)
- Contact:
- Funding:
Helmholtz Young Investigator Group
sprungmarken_marker_4427
Overview
Our research aims to solve complex problems of our time, focusing on sustainability and especially the energy transition and the power grid. Methodologically, the group “Data-driven analysis of complex systems (DRACOS)” combines exploratory data analysis, physical modeling and machine learning methods. One focus here is on the interpretability of the models: Instead of “black box” predictions, we develop transparent models.
Research
Mitigation of climate change requires a fundamental transformation of our energy system. Power plants based on fossil fuels must be replaced by renewable power sources, such as wind and solar power. This energy transition (“Energiewende”) towards a sustainable energy system raises numerous complex challenges, as power generation becomes more uncertain, while simultaneously more operational data becomes available. Hence, data-driven approaches become feasible and even necessary to fully understand the energy systems of today and tomorrow across all scales.
Machine learning and artificial intelligence can handle these enormous amounts of data but need to do so in a transparent way. Obtaining classifications or forecasts without explanations limits their use severely. Hence, we aim to explain “black box” models using interpretability tools or develop outright interpretable models. For example, an algorithm should explain which external factors, such as the feed-in of photovoltaic systems, the current electricity price or the time of day, are relevant for its prediction of the power grid frequency or household consumption. This transparency then enables synergies from machine and human models: Where is the machine better than the human? What can we learn from this for our human models and thus make them better?
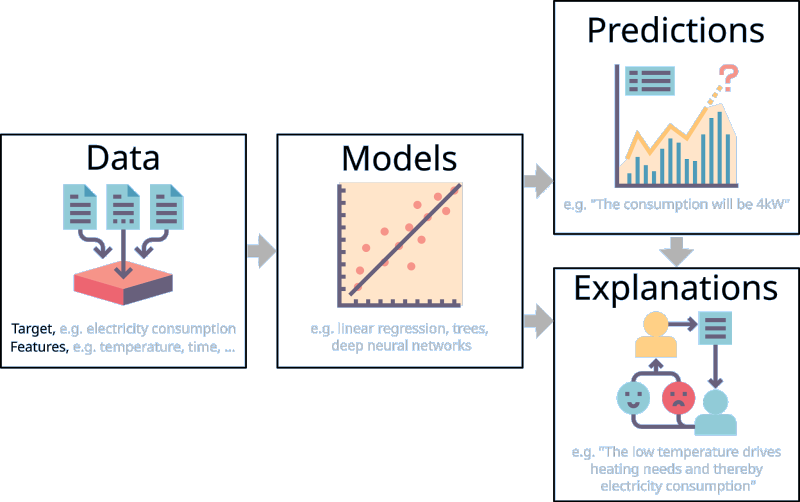
Our contributions are summarized in our vision and mission statements:
Vision: Enable a sustainable and collaborative future, where all energy generation is sustainable, data informs decisions and models are understood by everyone.
Mission: We develop and apply state-of-the-art machine learning methods to understand complex energy systems from households to transmission systems. We emphasize the openness of data and interpretability of models to enable collaboration among scientists and industry. We engage the general public by sharing and explaining our results.
Applications and partner
In our research, we utilize data from the Energy Lab and frequency measurements of various power grids. We collaborate with colleagues at KIT, other universities and Helmholtz centers, e.g. within Helmholtz AI (Helmholtz Artificial Intelligence Cooperation Unit).
For further information, contact Benjamin Schäfer.
Portrait | Name | Function/Research Interests |
---|---|---|
Xinyi Wen | PhD student (statistical inference, forecasting) | |
TT-Prof. Dr. Benjamin Schäfer | Assistant Professor (with tenure-track) | |
Sebastian Pütz, M.Sc. | PhD Student (Forecasting, Graph Neural Networks) | |
Ulrich Jakob Oberhofer, M.Sc. | PhD student (Stochastic Modelling, Physics-inspired Machine Learning) | |
Dr. Xiao Li | Postdoc position (AI for science) | |
Dr. Qiong Huang | Postdoctoral Researcher (Reinforcement Learning, Multi-agent Systems) | |
Hadeer Ahmed Hamed El Ashhab | PhD Student (Deep Learning and Forecasting) | |
Hallah Shahid Butt | PhD Student (Explainable Reinforcement Learning) |
Publications
Turowski, M.; Heidrich, B.; Weingärtner, L.; Springer, L.; Phipps, K.; Schäfer, B.; Mikut, R.; Hagenmeyer, V.
2024. Renewable and Sustainable Energy Reviews, 206, 114842. doi:10.1016/j.rser.2024.114842
Wen, X.; Oberhofer, U.; Gorjão, L. R.; Yalcin, G. C.; Hagenmeyer, V.; Schäfer, B.
2024. The 15th ACM International Conference on Future and Sustainable Energy Systems, 408–414, Association for Computing Machinery (ACM). doi:10.1145/3632775.3661944
Pütz, S.; El Ashhab, H.; Hertel, M.; Mikut, R.; Götz, M.; Hagenmeyer, V.; Schäfer, B.
2024. e-Energy ’24: Proceedings of the 15th ACM International Conference on Future and Sustainable Energy Systems, 447–453, Association for Computing Machinery (ACM). doi:10.1145/3632775.3661963
Butt, H. S.; Schafer, B.
2024. Proceedings of the 2024 Workshop on Explainability Engineering, 26–30, Association for Computing Machinery (ACM). doi:10.1145/3648505.3648510
He, H.; Boehringer, T.; Schäfer, B.; Heppell, K.; Beck, C.
2024. Scientific Reports, 14 (1), Art.-Nr.: 21288. doi:10.1038/s41598-024-72084-w
Hertel, M.; Pütz, S.; Schäfer, B.; Mikut, R.; Hagenmeyer, V.
2024. Helmholtz Artificial Intelligence Conference (Helmholtz AI 2024), Düsseldorf, Germany, June 12–14, 2024
Trebbien, J.; Pütz, S.; Schäfer, B.; Nygård, H. S.; Gorjão, L. R.; Witthaut, D.
2023. Powering solutions for decarbonized and resilient future smartgrids, 5 S., Institute of Electrical and Electronics Engineers (IEEE). doi:10.1109/ISGTEUROPE56780.2023.10407112
Kruse, J.; Cramer, E.; Schäfer, B.; Witthaut, D.
2023. PRX energy, 2 (4), Art.-Nr.: 043003. doi:10.1103/PRXEnergy.2.043003
Oberhofer, U.; Gorjao, L. R.; Yalcin, G. C.; Kamps, O.; Hagenmeyer, V.; Schäfer, B.
2023. 2023 IEEE Belgrade PowerTech, 1, Institute of Electrical and Electronics Engineers (IEEE). doi:10.1109/PowerTech55446.2023.10202986
Böttcher, P. C.; Schäfer, B.; Kettemann, S.; Agert, C.; Witthaut, D.
2023. Physical Review Research, 5 (3), Art-Nr.: 033139. doi:10.1103/PhysRevResearch.5.033139
Trebbien, J.; Rydin Gorjão, L.; Praktiknjo, A.; Schäfer, B.; Witthaut, D.
2023. Energy and AI, 13, Article no: 100250. doi:10.1016/j.egyai.2023.100250
Pütz, S.; Schäfer, B.
2023, June 28. 14th ACM International Conference on Future Energy Systems (e-Energy 2023), Orlando, FL, USA, June 20–23, 2023. doi:10.1145/3599733.3606298
Pütz, S.; Kruse, J.; Witthaut, D.; Hagenmeyer, V.; Schäfer, B.
2023. Companion Proceedings of the 14th ACM International Conference on Future Energy Systems (e-Energy ’23), 26–31, Association for Computing Machinery (ACM). doi:10.1145/3599733.3600247
Schäfer, B.; Rydin Gorjão, L.; Yalcin, G. C.; Förstner, E.; Jumar, R.; Maass, H.; Kühnapfel, U.; Hagenmeyer, V.
2023. (H. Sayama, Ed.) Complexity, 2023, Art.-Nr.: 2657039. doi:10.1155/2023/2657039
Lund Onsaker, T.; Nygård, H. S.; Gomila, D.; Colet, P.; Mikut, R.; Jumar, R.; Maass, H.; Kühnapfel, U.; Hagenmeyer, V.; Schäfer, B.
2023. Journal of Physics: Complexity, 4 (1), Article no: 015012. doi:10.1088/2632-072X/acbd7f
Hertel, M.; Beichter, M.; Heidrich, B.; Neumann, O.; Schäfer, B.; Mikut, R.; Hagenmeyer, V.
2023. Energy Informatics, 6 (S1), Art.-Nr.: 20. doi:10.1186/s42162-023-00278-z
Hertel, M.; Beichter, M.; Heidrich, B.; Neumann, O.; Ott, S. M.; Schäfer, B.; Mikut, R.; Hagenmeyer, V.
2023. Helmholtz Artificial Intelligence Conference (Helmholtz AI 2023), Hamburg, Germany, June 12–14, 2023
He, H.; Schäfer, B.; Beck, C.
2022. Scientific Reports, 12 (1), Artikel-Nr.: 12215. doi:10.1038/s41598-022-16109-2
Schäfer, B.; Beck, C.; Rhys, H.; Soteriou, H.; Jennings, P.; Beechey, A.; Heppell, C. M.
2022. Scientific Reports, 12 (1), Art.-Nr.: 12346. doi:10.1038/s41598-022-16342-9
Kruse, J.; Schäfer, B.; Witthaut, D.
2022. Electric Power Systems Research, 212, Art.-Nr.: 108489. doi:10.1016/j.epsr.2022.108489
Lund Onsaker, T.; Nygård, H. S.; Gomila, D.; Colet, P.; Mikut, R.; Jumar, R.; Kühnapfel, U.; Maass, H.; Hagenmeyer, V.; Witthaut, D.; Schäfer, B.
2022, September 27. doi:10.48550/arXiv.2209.15414
Hertel, M.; Ott, S.; Neumann, O.; Schäfer, B.; Mikut, R.; Hagenmeyer, V.
2022. 36th Annual Conference on Neural Information Processing Systems (NIPS 2022), Online, November 28–December 9, 2022
Böttcher, P. C.; Rydin Gorjão, L.; Beck, C.; Jumar, R.; Maass, H.; Hagenmeyer, V.; Witthaut, D.; Schäfer, B.
2022. Energy Advances, 2 (1), 91–97. doi:10.1039/D2YA00150K
Hertel, M.; Ott, S.; Schäfer, B.; Mikut, R.; Hagenmeyer, V.; Neumann, O.
2022. Proceedings - 32. Workshop Computational Intelligence: Berlin, 1. - 2. Dezember 2022. Hrsg.: H. Schulte, F. Hoffmann; R. Mikut, 93–110, KIT Scientific Publishing
Schäfer, B.; Pesch, T.; Manik, D.; Gollenstede, J.; Lin, G.; Beck, H.-P.; Witthaut, D.; Timme, M.
2022. doi:10.48550/arXiv.2209.13278
Schäfer, B.; Pesch, T.; Manik, D.; Gollenstede, J.; Lin, G.; Beck, H.-P.; Witthaut, D.; Timme, M.
2022. Nature Communications, 13 (1), Art.-Nr.: 5396. doi:10.1038/s41467-022-32917-6
Schmidt, R.; Haehne, H.; Hillmann, L.; Casadiego, J.; Witthaut, D.; Schafer, B.; Timme, M.
2022. IEEE Access, 10, 76682–76692. doi:10.1109/ACCESS.2022.3191665
Heidrich, B.; Mannsperger, L.; Turowski, M.; Phipps, K.; Schäfer, B.; Mikut, R.; Hagenmeyer, V.
2022. DACH+ Conference on Energy Informatics German Federal Ministry for Economic Affairs and Energy
Heidrich, B.; Mannsperger, L.; Turowski, M.; Phipps, K.; Schäfer, B.; Mikut, R.; Hagenmeyer, V.
2022. Energy Informatics, 5 (S1), Article no: 20. doi:10.1186/s42162-022-00214-7
Anvari, M.; Proedrou, E.; Schäfer, B.; Beck, C.; Kantz, H.; Timme, M.
2022. Nature Communications, 13 (1), Art.-Nr.: 4593. doi:10.1038/s41467-022-31942-9
Cramer, E.; Gorjao, L. R.; Mitsos, A.; Schafer, B.; Witthaut, D.; Dahmen, M.
2022. IEEE Access, 10, 8194–8207. doi:10.1109/ACCESS.2022.3141875
Tchuisseu, E. B. T.; Dongmo, E.-D.; Procházka, P.; Woafo, P.; Colet, P.; Schäfer, B.
2022. European Journal of Applied Mathematics, 34 (3), 467–483. doi:10.1017/S095679252100036X
Rydin Gorjao, L.; Vanfretti, L.; Witthaut, D.; Beck, C.; Schäfer, B.
2022. IEEE Access, 10, 18065–18073. doi:10.1109/ACCESS.2022.3150338
Rydin Gorjão, L.; Jumar, R.; Maass, H.; Hagenmeyer, V.; Yalcin, G. C.; Kruse, J.; Timme, M.; Beck, C.; Witthaut, D.; Schäfer, B.
2020. Nature Communications, 11 (1), Art.-Nr. 6362. doi:10.1038/s41467-020-19732-7