Phase Transition Analysis in Battery Materials Powered by POLiS AI Tools
- Contact:
- Project Group:
Machine Learning for High-Throughput Methods and Mechatronics (ML4HOME)
Research on phase transitions is of great importance for the development of novel battery electrode materials.
Researchers seek active materials with superior electrochemical properties and aim to fine-tune material compositions to reliably synthesize these materials.
Modern operando XRD platforms are capable of analyzing the crystal structure of these materials during experiments, and are able to autonomously generate hundreds of XRD scans.
Analysis of these scans usually requires manual intervention and can therefore bottleneck research processes.
This project aims to support material scientists by detection of similarity regions in phase diagrams.
We provide a web application which utilizes dimensionality reduction using variational auto-encoder neural networks combined with various clustering algorithms to group XRD patterns.
This enables researchers to gain an overview of their samples and see phase transitions, without significantly altering their workflow.
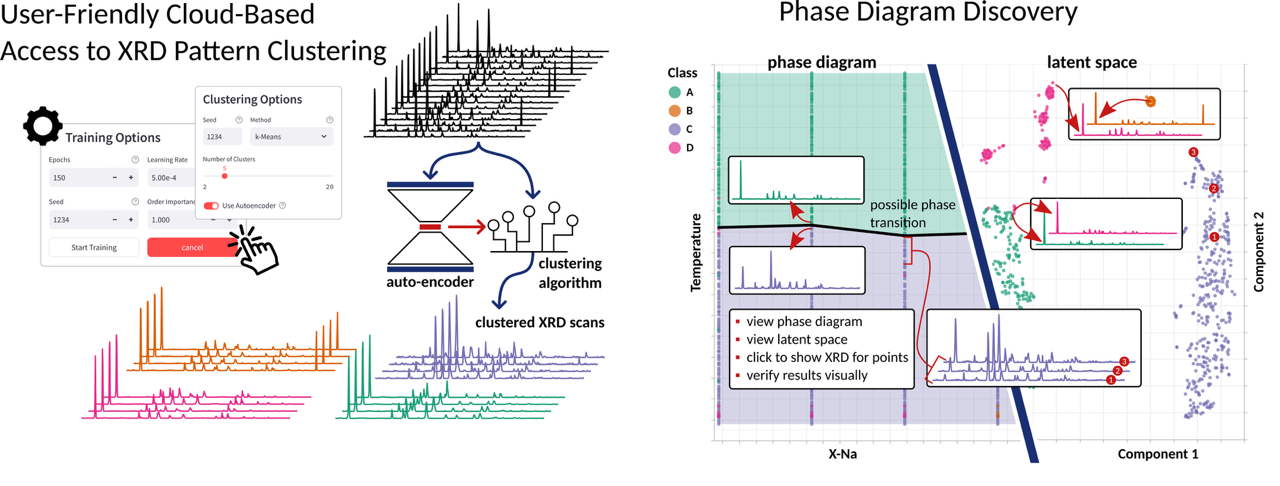